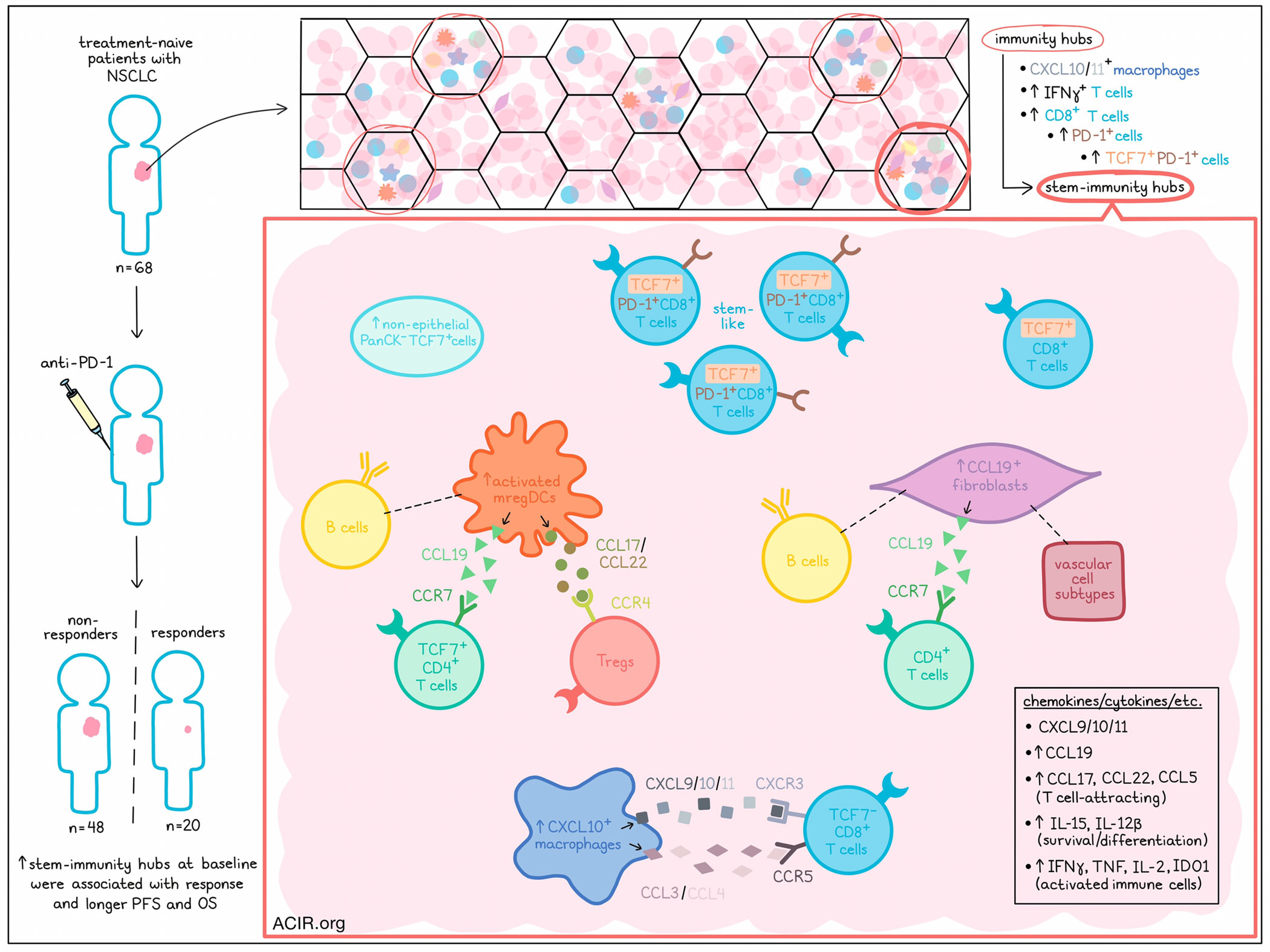
Chen, Nieman, and Spurrell et al. recently evaluated multicellular “immunity hubs” in samples of human non-small cell lung cancer (NSCLC) taken prior to treatment with anti-PD-1. Using imaging techniques and spatial transcriptomics, the researchers identified and characterized novel “stem-immunity hubs” that were strongly associated with PD-1 blockade responses and outcomes. Their results were published in Nature Immunology.
Based on past evidence showing that immunity hubs – neighborhoods around CXCL10/11+ cells – were associated with response to anti-PD-1 immunotherapy in other cancers, Chen, Nieman, and Spurrell et al. investigated their role in NSCLC by evaluating specimens from 68 treatment-naive patients. To visualize immunity hubs, the researchers used single-molecule fluorescence in situ hybridization (smFISH) based on CXCL10/11 (T cell-attracting chemokines), CD3E (T cell marker), IFNG (T cell activation marker), CXCL13 (tumor-reactive T cell marker), PanCK (epithelial marker) and DAPI (nuclear marker). This revealed a total of 3777 immunity hubs across all samples. Analysis of the results showed a 5.5-fold increase in the median frequency of immunity hubs in anti-PD-1 responders (n=20) relative to non-responders (n=48).
Immunity hubs were enriched for T cells expressing IFNγ. Further analysis of T cell states among 2712 immunity hubs identified using multiplex immunohistochemistry showed that relative to other areas of the tumor, immunity hubs harbored more CD8+ T cells, including activated PD-1+ cells and stem-like TCF7+PD-1+ cells. Unsupervised clustering identified 7 immunity hub subclusters, and the researchers found that the fraction of area occupied by one subcluster, subcluster 3, was increased 106-fold in responders vs. non-responders. Further, patients with subcluster 3 representing at least 0.1% of total area experienced better progression-free and overall survival relative to patients below this threshold. Two non-responding patients also had a high frequency of subcluster 3, though a closer look revealed that both patients showed evidence of good responses outside of progression that had occurred in the central nervous system.
Evaluating which cell types were enriched in each immunity hub subcluster, the researchers found that while subcluster 1 was enriched for T cells that expressed IFNγ and were proliferative, subcluster 3 had increased non-epithelial PanCK-TCF7+ cells and TCF7+ CD8+ T cells, and were particularly enriched for stem-like TCF7+PD1+ CD8+ T cells, which were uncommon in other parts of the tumor. Further, a higher fraction of TCF7+PD1+ CD8+ T cells were found within subcluster 3 in responders than non-responders, and imaging confirmed that they were densely aggregated. Based on this evidence, subcluster 3 immunity hubs were deemed as “stem-immunity hubs”. While CXCL10/11 and stem-like TCF7+PD1+ CD8+ T cells were both independently correlated with response to anti-PD-1, immunity hubs containing both CXCL10/CXCL11 expression and TCF7+ cells showed stronger correlations with response than either feature alone, suggesting the importance of cellular niches.
Next, the researchers used MERFISH – an imaging technology that measures gene expression using RNA probes with barcoded tails – to detect 479 genes marking various immune, stromal, and epithelial cell states at the single-cell level. In 4 samples with CXCL10/11 expression and a large tissue area, taken from patients who went on to respond to anti-PD-1, the researchers divided the tissue into “tiles”, which were designated as stem immunity hubs, non-immunity hubs, vascular hubs, and other tumor tissue. Analyzing chemokines, they found that in addition to expressing genes for CXCL9/10/11, stem-immunity hubs expressed genes for CCL19 and its receptor, CCR7; various T cell-attracting chemokines, including CCL17, CCL22, and CCL5; IL-15 and IL-12B, associated with survival and differentiation; and IFNγ, TNF, IL-2, and IDO1, consistent with the high density of activated immune cells. Looking at other cell types, the researchers found that while stem-immunity hubs were depleted of epithelial cells and SPP1+ macrophages (associated with fibrosis and poor outcomes), they were enriched for mregDCs and CXCL10+ macrophages.
Investigating any preferential cell–cell interactions within stem-immunity hubs, the researchers found that mregDCs were most frequently adjacent to TCF7+ CD4+ T cells and Tregs, suggesting on-site CD4+ T cell activation as cytotoxic effectors, helper T cells, or Tregs. Spatial ligand–receptor analysis led to inferences that activated mregDCs utilized the CCL17/22–CCR4 axis to interact with Tregs, as well as the CCL19–CCR7 axis to interact with TCF7+ CD4 T cells. Additionally, the researchers found that CXCL10+ macrophages preferentially interacted with TCF7- CD8+ T cells, both within and outside of stem-immunity hubs, via the CXCL9/10/11–CXCR3 and CCL3/4–CCR5 axes, consistent with their phenotypes.
Next, the researchers parsed out stromal populations into pericytes, smooth muscle cells, three subtypes of endothelial cells, and four subtypes of fibroblasts. One fibroblast subcluster in particular was highly enriched for CCL19 expression, and these CCL19+ fibroblasts were significantly enriched in stem-immunity hubs. Like CCL19-expressing mregDCs, these fibroblasts colocalized with B cells and CD4+ T cells. However, while mregDCs associated with Tregs, CCL19+ fibroblasts colocalized with various vascular cell subtypes. CCL19+ fibroblasts were found to interact preferentially with CD4+ T cells via the CCL19-CCR7 axis, suggesting fibroblast-mediated recruitment of CD4+ T cells into stem-immunity hubs.
Finally, comparing stem-immunity hubs to mature tertiary lymphoid structures (TLSs), Chen, Nieman, and Spurrell et al. found that unlike TLSs, stem-immunity hubs were compositionally distinct, and notably lacking in B cell follicles. Evaluating differentially expressed genes between stem-immunity hubs and TLSs, the team found that stem immunity hubs were strongly enriched for the IFNγ-response gene sets, including CXCL10 and CXCL9, as well as for markers of activated CD8+ T cells and macrophages. TLSs on the other hand, were enriched for B cell genes and markers of less differentiated lymphocytes.
Together, these results identify stem-immunity hubs, marked by TCF7+PD1+ CD8+ T cells, activated mregDCs, CCL19+ fibroblasts, and various chemokines, as intratumoral multicellular niches indicative of ongoing, active immunity. These stem-immunity hubs and the preferential cell–cell interactions that occur within them can contribute to or predict responses to PD-1 blockade in patients with NSCLC. Better understanding these hubs could be used to optimize treatment selection in patients, and could potentially be targeted by immunotherapies in the future.
Write-up and image by Lauren Hitchings
Meet the researcher
This week, co-first author Jonathan Chen answered our questions.
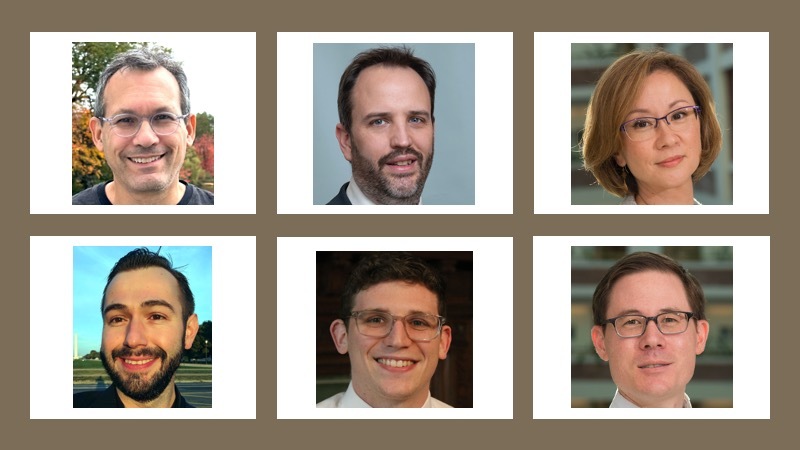
What was the most surprising finding of this study for you?
We were surprised by the level of organization within these hubs. We pathologists have been looking at lung cancer tissue sections for generations using H&E, but the hubs were passed over because they just look like a jumble of lymphocytes, myeloid cells, and tumor cells. However, when we employ advanced RNA ISH microscopy to look at specific genes in these cell types, the biology pops out. Even within the hubs, there is substructure; certain kinds of cells preferentially interact with one another.
What is the outlook?
The most obvious implication of our work is that we can use the presence of the immunity hub, particularly the stem-immunity hub subclass, as a biomarker to predict response to immunotherapy. Our finding that immune cells are organized in small regions within tumors shows that there are positive feedback loops between cells, likely mediated by chemokines and cytokines, that aggregate cells, at least transiently. Our next step is to understand how this happens.
We think the immunity hub may be how the immune system organizes to fight tumors. Therefore, we may be able to develop therapeutics based on findings in this study to augment immunity hubs and support the antitumor immune response. In particular, stem-immunity hubs may be sites where stem-like T cells are nurtured, and could serve as platforms for launching a durable antitumor response.
What was the coolest thing you’ve learned (about) recently outside of work?
The James Webb Space Telescope found a huge galaxy that formed not too long after the Big Bang. This is interesting because the presence of such large galaxies is not possible based on current models. So, this observation might force us to rethink our theory of the origins of the universe.