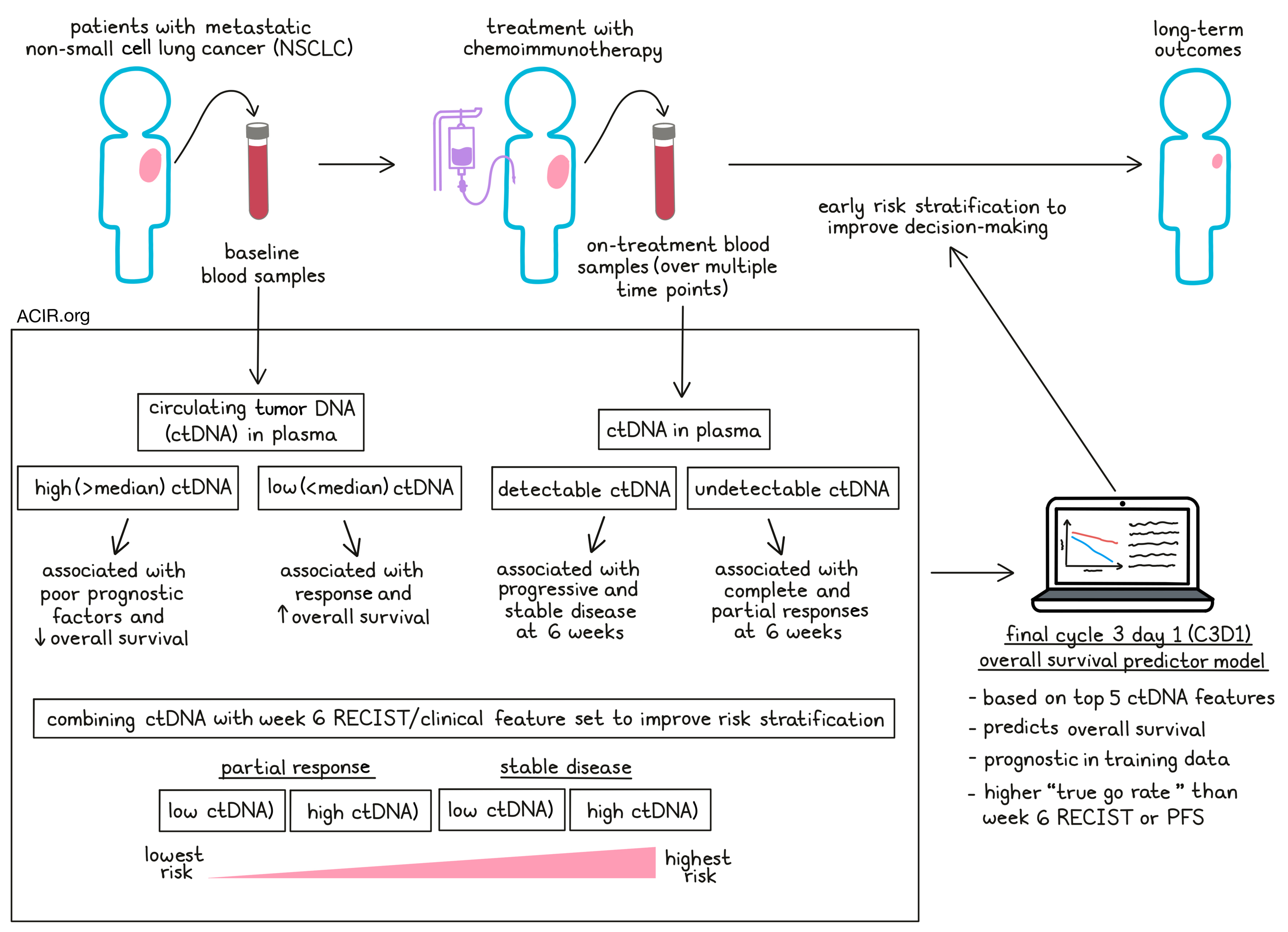
It remains a challenge to determine which patients might benefit from immunotherapy, and in drug trials, early endpoints, such as progression-free survival (PFS) and overall response rates, may not correspond with overall survival (OS). Therefore, trials may take many years to conduct to show a benefit in OS. Looking into other early measures of therapy response, Assaf et al. performed analyses on circulating tumor DNA (ctDNA) at baseline and dynamics during therapy to assess whether ctDNA dynamics could improve prediction models for immunotherapy response. Their results were recently published in Nature Medicine.
The researchers made use of samples and information from the registration-driven Impower150 trial, which led to the approval of a chemoimmunotherapy combination (including ICB with anti-PD-L1) in first-line non-small cell lung cancer. Baseline and on-treatment plasma samples were available from 466 patients. Deep targeted sequencing was performed on these samples and on patient PBMCs, and data were processed by a cell-free DNA computational pipeline to remove common germline and clonal hematopoiesis-derived mutations to identify tumor-derived somatic mutations. These corrections were important, as nearly 10% of patients switched from ctDNA-positive to ctDNA-negative after correction for PBMC variants. Baseline characteristics were similar between this subset of patients and the intention-to-treat population of the study.
On-treatment samples were used to track changes in ctDNA in response to treatment. Using bioinformatics, the on-treatment variant allele frequency for every mutation detected in the baseline samples was estimated. In total, 282 patients had plasma variants that were also detected in PBMCs (considered germline or CHIP mutations), and 45 patients switched from ctDNA-positive to -negative after correction for PBMC variants.
At baseline, ctDNA was detected in 393 patients, of which 89% had pathogenic alterations. For further analyses, the population was split into a training (n=240) and a test set (n=226), which had similar survival outcomes, baseline clinical characteristics, and ctDNA status, and the treatment arms were similarly distributed among these sets.
In the training set, the baseline ctDNA prevalence was 85%, which decreased on treatment to 79.3% (at cycle 2, day 1[C2D1]), 77.0% (C3D1), 77.3% (C4D1), and 76.4% (C8D1). Baseline ctDNA was predictive for outcomes. The patients with ctDNA positivity could then be further stratified based on the median ctDNA level, with those with levels above the median having a shorter OS than those with levels below the median. Higher levels were also associated with poor prognostic characteristics such as age below 65, history of smoking, baseline tumor size, and the number of metastatic sites.
Treatment correlated with a reduction in ctDNA levels, and treatment RECIST responses at week 6 were associated with ctDNA levels. Patients with complete response (CR) or partial response (PR) had lower ctDNA levels for all on-treatment time points than those with stable disease (SD) or progressive disease (PD). ctDNA levels also correlated with tumor size at the baseline and C3D1 time points. When early radiographic tumor assessments were used to predict outcomes, patients with SD at week 6 had a shorter OS than those who experienced PR. ctDNA data at a similar time point showed a shorter OS for patients with detectable ctDNA levels. Combining the ctDNA data with the RECIST by radiographic tumor assessments could further improve the risk stratification, and patients with SD could be split into SD/ctDNA high risk vs. SD/ctDNA low risk; a similar split could be made for patients with PR.
The researchers then investigated various metrics to describe ctDNA dynamics. Modeling these together in a machine learning framework, PFS and OS were predicted from each plasma collection time point. Models were then trained for each time point, with all measurements from baseline until that time point included. The model performance was best for OS at the C3D1 and C8D1 time points. Given that limited samples from C8D1 were available, the OS model was focused on the C3D1 time point. The model was compared to clinical features alone or combined with ctDNA features. The combined ctDNA and clinical feature set performed better than the clinical feature set alone.
To reveal the top features of the C3D1 OS ctDNA model, the ctDNA features that were chosen during training in >50% of cross-validations and which had a positive gain metric were selected. The final model was then fit using the top five identified ctDNA features. Patients were grouped based on the C3D1 OS model predictions into high-, intermediate-, and low-risk groups. Survival analyses confirmed that these three groups were prognostic in the training data. In the test set, the high-risk group also had a shorter OS than those with intermediate and low risk, and patients with SD and PR could be stratified into high-risk and low–intermediate-risk groups.
Further validation was done using an external patient cohort from the OAK clinical trial. This dataset also included patients treated in the second-line setting, and included patients treated with different treatment regimens. The C3D1 OS predictor model could also effectively identify patients with high-risk and low-intermediate risk in this cohort.
To see if ctDNA could be used as an early endpoint for decision-making in early phase II trials, 2,000 simulations of 30 patients per arm from the Impower150 test data samples were conducted. Then the proportion of simulation where the active arm had higher rates of response were compared to the control arm (“true go rate”) using various early endpoints. ctDNA response alone had higher true go rates than week 6 RECIST or PFS data. When the ctDNA data were combined with the RECIST outcomes or PFS, the true go rate improved.
In conclusion, this research shows that ctDNA levels in plasma during immunotherapy treatment can be used as an early endpoint in clinical trials to predict outcomes, and is particularly predictive when combined with RECIST response rates. Therefore, it may be beneficial for immunotherapy trials to include ctDNA plasma testing to allow early risk stratification and decision-making.
Write-up by Maartje Wouters, image by Lauren Hitchings
Meet the researcher
This week, first author Zoe June Assaf and lead authors David Shames and Katja Schulze answered our questions.
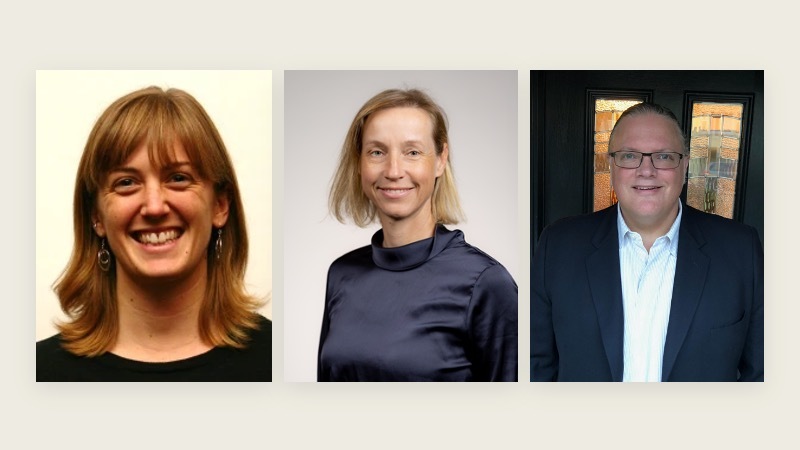
What was the most surprising finding of this study for you?
Circulating tumor DNA (ctDNA) technology is positioned to transform patient management by providing real-time assessments of a patient’s molecular tumor burden using a simple blood draw. There are diverse approaches used in the literature to summarize ctDNA levels and integrate ctDNA features for association with clinical outcomes, as well as the open question regarding which on-treatment time points may be optimal for longitudinal ctDNA analyses.
The author team did a thorough assessment at the beginning of the study to determine the most suitable ctDNA assay for addressing these important questions. We were quite surprised how well the model developed in IMpower150 validated in the OAK study. It impressively stratified patients using the model risk thresholds, despite the fact that different assays were used for ctDNA testing in both studies.
What is the outlook?
The next steps for this approach will be to prospectively evaluate the technology as a decision-making tool for clinical development, and also to better understand how broadly applicable the algorithm is across ctDNA technologies, as well as other approaches for liquid biopsy analysis.
What was the coolest thing you’ve learned (about) recently outside of work?
California has received a lot of precipitation over these last few winter months while temperatures were also quite low. It was stunning flying over snow-covered mountains in the Bay Area and hearing about backcountry skiers conquering Mt. Diablo near Walnut Creek. A truly rare and beautiful sight.