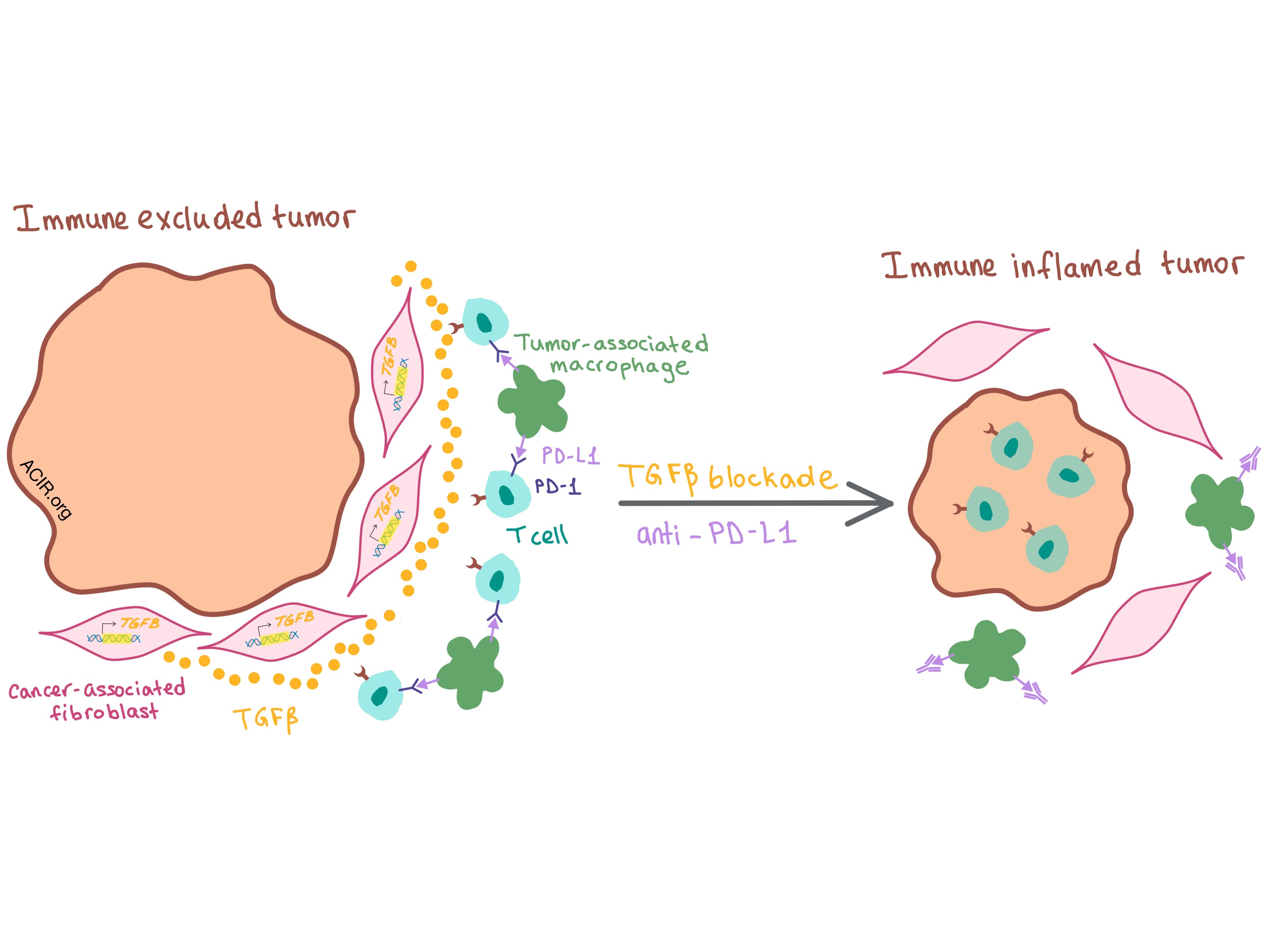
Trying to figure out why some patients respond to immune checkpoint blockade while others do not may sometimes feel as hopeless as repeatedly hitting one’s head against a brick wall. However, the latest research by Mariathasan et al. and Tauriello et al., published in Nature, may tone down the headache. While the two groups examined different cancer types and utilized different approaches, they came to the same conclusion: for a subset of situations, TGFβ predicts poor response, and its inhibition allows PD-L1 blockade to do its job.
Mariathasan et al. examined pre-treatment samples from a large cohort of patients with metastatic urothelial cancer (mUC) treated with atezolizumab (a PD-L1 inhibitor) in a phase II clinical trial in order to understand which biomarkers correlated with response. Using immunohistochemistry, transcriptome RNA sequencing, and gene set enrichment analysis, they found that three biological pathways and interactions between them appeared to determine response outcome. Pre-existing T cell immunity (CD8+ T-effector cells [Teff], IFNγ expression, PD-L1 expression on immune cells), high tumor mutation burden (TMB), and predicted neoantigen burden correlated with response, while TGFβ signaling correlated with non-response.
Whether all three pathways were relevant as predictors of response depended on the tumor phenotype. Human solid tumors generally fall into one of three phenotypes: immune inflamed (T cells infiltrating the tumor), immune excluded (T cells restricted to the stromal tissue surrounding the tumor), and immune desert (T cells found in neither the tumor center nor surrounding stroma). The mUC cohort in this study exhibited all three phenotypes: half the tumors were excluded, and the other half was evenly split between inflamed and desert. While TMB was associated with anti-PD-L1 response in both excluded and inflamed tumors, CD8+ Teff signature was only relevant in inflamed tumors, as CD8+ T cells were absent from the immune excluded tumors. The physical separation of T cells from tumor cells in excluded tumors and the proximal stromal tissue rich in fibroblasts led the investigators to focus on a pan-fibroblast TGFβ response signature (F-TBRS) as a biomarker; interestingly, they found it to be associated with non-response only in excluded tumors.
These results led the researchers to hypothesize that TGFβ pathway activation in stromal fibroblasts within the TME resulted in the physical exclusion of T cells from the tumor, thus inhibiting response to PD-L1 blockade. Turning to the mouse to directly test this hypothesis, the researchers found that in the murine EMT6 mammary carcinoma and MC38 colorectal cancer (CRC) models, both of which exhibit immune excluded phenotype, blockade of either PD-L1 or TGFβ alone had little effect, but combination of the two antibodies led to a significant reduction in tumor burden in a CD8+ T cell-dependent manner. Combination therapy reduced TGFβ signaling (especially in non-immune cells, such as fibroblasts) and increased T cell infiltration into the tumor, changing the T cell distribution within the TME — T cells moved away from the stromal border and closer to tumor center. Thus, inhibition of both TGFβ and PD-L1 converted tumors from excluded to inflamed phenotype, supporting a model where TGFβ signaling interferes with antitumor immunity by restricting the movement of T cells within the TME.
In a separate study, Tauriello et al. analyzed the relationship between genetic mutations and the TME in CRC. They used a driver (designated as L) to target mutations affecting up to four different oncogenic pathways (the Apc, Kras, Tgfbr2, and Trp53 genes, designated as A, K, T, and P, respectively) to intestinal stem cells in CRC mouse models. The researchers found that LAKTP mice exhibited an immune excluded phenotype that tended to develop metastases. These mice had highly active TGFβ signaling in the tumor stroma as well as high expression of two cancer-associated fibroblast (CAF) TGFβ-induced genes, which are predictors of poor prognosis in humans. This genetic phenotype was representative of the TME in advanced human CRC.
RNA-sequencing of flow-sorted cell populations confirmed CAFs as the main source of TGFβ. Using the TGFBR1-specific inhibitor galunisertib decreased TGFβ response signature in CAFs and T cells, inhibited TGFβ signaling in the TME, and resulted in reduced primary tumor volume and decreased metastatic burden in a manner that was dependent on CD8+ cytotoxic T lymphocytes (CTLs) and CD4+ T-helper (TH) cells. Most treated mice rejected a rechallenge tumor.
In mice with more severe metastatic disease, however, galunisertib reduced metastatic burden but rarely led to complete remission. This was due to increased expression of PD-L1 on stromal cells (mostly tumor-associated macrophages) that were recruited as the metastases grew, as well as high PD-1 expression on activated T cells. While anti-PD-L1 therapy alone exerted only a minor therapeutic effect, combination of anti-PD-L1 and galunisertib increased lymphocyte infiltration, enhanced the production of T-bet and IFNγ in CD4+ TH cells and granzyme B in CTLs, and led to metastasis eradication and durable recurrence-free survival. These experiments indicate that, similar to the results observed by Mariathasan et al., combination treatment switched the tumor phenotype from excluded to inflamed.
To confirm clinical relevance, Tauriello et al. analyzed human microsatellite-stable CRC samples and found that a substantial subset of patients had reduced T cell differentiation (from naive to TH1) and enhanced TGFβ and CAF gene expression, which correlated with poor prognosis and predicted disease relapse. The authors conclude that the increased levels of TGFβ in the TME of CRC restricts the adaptive immune response by excluding CTLs from the tumor and inhibiting TH1-effector cell phenotype.
In summary, both studies demonstrate that blockade of TGFβ signaling allows for lymphocyte infiltration into the tumor, converts the TME to a more inflamed state, and makes it susceptible to PD-1/PD-L1 checkpoint blockade, thus supporting clinical translation.
by Anna Scherer