In recent years, researchers have made much progress in understanding the therapeutic response to checkpoint blockade strategies, but to further improve the success rate, clues might be found in a deeper investigation of the mechanisms behind the response. In a recent publication in Cancer Cell, Grasso et al. describe their analysis of a large number of prospective biopsy samples from metastatic melanoma patients treated with anti-PD-1 (Nivolumab) or anti-PD-1 combined with anti-CTLA-4 (Ipilimumab) as part of the Checkmate 038 trial. Transcriptome analysis by bulk RNAseq, with modeling and validation of pathways of interest in 58 human melanoma cell lines, could then be correlated with clinical response to uncover these mechanism-based clues.
From 101 patients, 84 baseline and 85 on-therapy samples were subjected to RNAseq. Of these, 68 samples were paired, and for the unpaired samples, an optimal pooled t-test was applied to enable comparisons before and after treatment. Clinical response was defined as complete or partial response (CRPR), while non-responders had stable disease (SD) or progressive disease (PD).
The researchers first analyzed the immune infiltrate using denominated microenvironment cell populations-counter (MCP-counter), which deconstructs RNAseq data to define immune cell types. Patients responding to therapy had higher baseline levels of T cells, B lineage cells, myeloid dendritic cells, and NK cells than non-responders. In general, all patients had increases in T cell infiltration upon therapy, but the changes were most extensive in responders. Principal component analysis confirmed that the most predictive factor was the degree of T cell infiltration in both the baseline and the on-therapy samples. Furthermore, t-SNE embedding to cluster the genes with their closest immune cell signature derived from MCP-counter resulted in two gene clusters; one that was correlated with the T cell score, and one that was anti-correlated, further confirming the importance of T cell infiltrate for therapy response.
Given the clear predictive value of T cell infiltration, the researchers then studied the drivers behind this response by examining effector cytokines and toxic granules associated with T cell activity. They found a higher expression of genes encoding perforin, granzyme B, TRAIL, and TNFα in responders. These expression patterns followed the expression of IFNγ, which led them to hypothesize that cancer cell expression of interferon response genes might be relevant for attracting immune cells and stimulating their function. This suggests that patients with a lack of response should have melanoma cells incapable of responding to IFNγ.
To study this hypothesis, Grasso et al. analyzed the transcriptome, before and after IFNγ treatment, of 57 previously established primary human melanoma cell lines, as well as the non-malignant melanocyte cell line HeMa. Upon IFNγ treatment, changes in IFNγ response genes were found in most cell lines. The responding cell lines had a strong upregulation of genes associated with the antigen processing and presentation machinery, increases in chemokines such as CXCL9, CXCL19, and CXCL11, and other genes in interferon signaling pathways. However, as anticipated, a distinct subset of 12 cell lines, characterized by JAK1 or JAK2 loss-of-function mutations, did not signal through the IFNγ receptor.
The researchers then went back to the biopsies and found that responders had a higher baseline expression of interferon response genes than non-responders, and that the expression further increased upon treatment. Within the group of samples from non-responders, there were even samples without expression of interferon response genes at baseline. The main differences between responders and non-responders were a higher expression in antigen processing and presentation pathway genes in responders, particularly in genes encoding HLA class I and II, B2M, TAP1, TAP2, NLRC5, and CIITA. To correct for the fact that these samples contained all cells in the microenvironment and not just tumor cells, the researchers normalized the data for LCA (CD45). After this correction, the HLA I score was not different in responders, suggesting that this signal was driven by immune cell infiltration. Therefore, these data should be validated by single cell RNAseq to determine the cell type driving these signatures.
The authors then looked at two pathways recently suggested to be associated with immune exclusion in the tumor microenvironment. First, they assessed WNT signaling by using a nine-gene RNAseq-based WNT score. Responders had a significant decrease in the score after therapy, while the score did not change in non-responders. Tracking the WNT score and T cell infiltration between baseline and therapy samples suggested a relatively random distribution in non-responders, while the responders trended from high WNT/low T cell to a low WNT/ high T cell infiltrate between before and on-therapy samples. The same trend of a lower WNT score after treatment with IFNγ was found in the cell lines, as well as downregulation of MYC, a target gene in the WNT signaling pathway.
Second, the researchers analyzed the recently developed ‘Jerby-Arnon’ immune exclusion signature; the main driver of that signature, CDK4; and PAK4, a gene recently found to be overexpressed in patients with low T cell infiltrates who do not respond to anti-PD-1 therapy. The signature and both genes were downregulated in responders – effects that were not found in the IFNγ-treated cell lines – suggesting these are likely downstream effects.
In summary, the results presented by Grasso et al. indicate changes in gene signatures related to immune infiltration in patients responding to checkpoint therapy. Enhanced IFNγ signaling, triggered by the activity of pre-existing T cells in the tumor, resulted in increases in genes related to the antigen processing and presenting pathways, and increases in chemokines, resulting in more immune cell attraction. This was further aided by a reduction in WNT signaling and downstream effects on genes of the ‘Jerby-Arnon’ immune exclusion signature, CDK4, and PAK4. Together, this achieved a more welcoming immune environment in responders.
The mechanisms behind the therapy response to checkpoint inhibition presented here could spark new research into potential combination treatment strategies. Raising interferon signaling in tumor cells using, for instance, oncolytic viruses that trigger IFNγ production or intratumoral TLR stimulation, may pave the way for improved outcomes to checkpoint blockade in patients who currently do not respond to therapy.
Write-up by Maartje Wouters, image by Lauren Hitchings
Meet the researcher
This week, first author Catherine Grasso answered our questions.
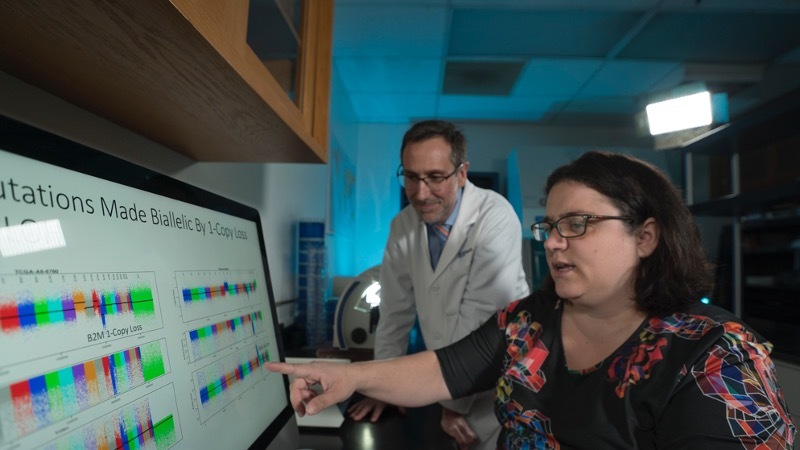
What prompted you to tackle this research question?
The initial motivation to tackle this problem was the observation that immune infiltration seemed to be a primary driver of response. We wanted to break down the samples and see if there were different responses for different immune cell types.
What was the most surprising finding of this study for you?
We looked at a cell line cohort to identify additional mutations, potentially in the interferon gamma pathway, driving resistance to interferon gamma, and we didn't find them. The ones we knew, B2M and JAK1/2, were present and we did not find more. With that said, we found that immune infiltration before treatment played a large role, and that the mutations or gene expression driving infiltration played a much larger role.
What was the coolest thing you’ve learned (about) recently outside of work?
It's possible to cure schizophrenia using immunotherapy.
https://www.nytimes.com/2018/09/29/opinion/sunday/schizophrenia-psychiatric-disorders-immune-system.html