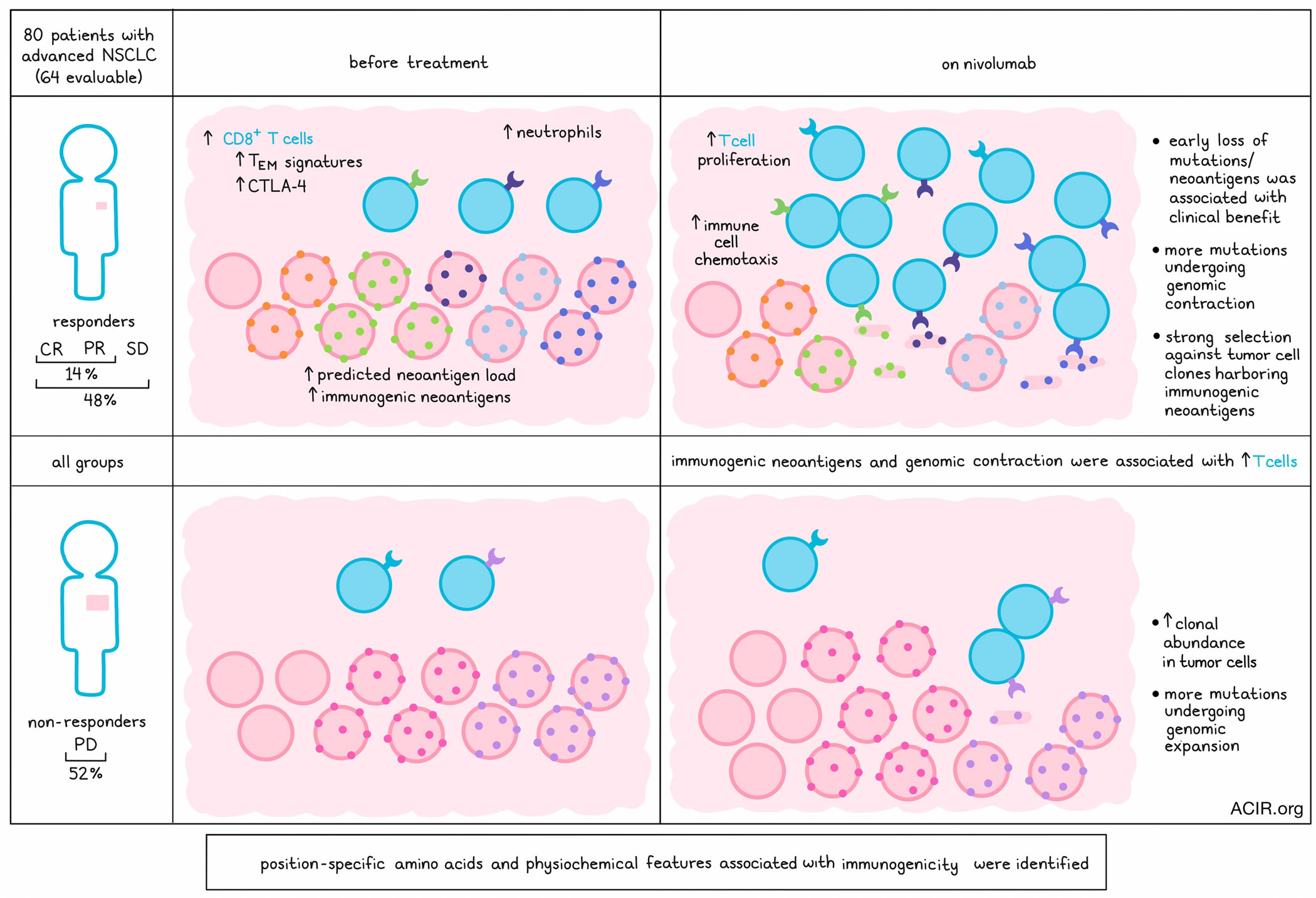
While it is known that neoantigen immunoediting plays a role in immune checkpoint blockade (ICB) efficacy, it is unclear how neoantigen recognition by T cells impacts tumor presentation of antigens in response to ICB. Alban and Riaz et al. evaluated changes in tumors in relation to neoantigen reactivity and therapy response to address whether ICB drives recognition of neoantigens. Their results were recently published in Nature Medicine.
Biopsy samples were obtained from 80 patients with advanced non-small cell lung cancer (NSCLC) progressing on chemotherapy. The biopsy samples were obtained from the same tissue site before and during nivolumab treatment. Sufficient material was available from 64 patients, and within this cohort, the complete response (CR) and partial response (PR) rate to nivolumab was 14%, while the rate of CR, PR, and stable disease (SD) was 48%.
RNAseq analysis followed by gene-set enrichment analysis (GSEA) showed increased CD8+ T cells, CTLA-4 expression, neutrophils, and effector memory T cell signatures pretreatment in those with SD and PR (observed in both low and high tumor mutational burden [TMB] subsets). Nivolumab treatment upregulated pathways related to immune cell chemotaxis and T cell proliferation.
Since there was increased immune activation in response to treatment, the researchers assessed changes to targets of the immune system. Comparing pretreatment and on-treatment whole exome sequencing (WES) data, 24.4% of single-nucleotide polymorphisms (SNVs) were found to be shared, but there was no strong link to therapy RECIST-based responses in terms of the overall net change in SNVs from pretreatment. The median change in SNVs at 3 weeks after treatment was, however, significantly associated with overall survival (OS) and progression-free survival (PFS), while changes in indels and indel-derived neoantigens were not associated with response. DNA mutational loss/gain was compared with transcriptional expression of variants, which revealed that variants increased with genomic expansion and decreased with genomic contraction. Pretreatment TMB was not associated with OS, while in silico predictions of neoantigen load had a strong trend toward longer survival.
The researchers assessed how changes in cancer cell fraction (CCF; the fraction of cancer cells carrying a given mutation), intratumoral heterogeneity, and tumor mutation clonality related to treatment outcomes. Samples with a higher number of mutations undergoing genomic contraction (CCF decrease ≤10%) were almost always responders or SD, while those with progressive disease (PD) were more likely to have genomic expansion (CCF increase ≥10%). Tumors from patients with SD or PR could undergo either contraction or expansion. Overall, increased genomic contraction trended toward improved OS.
To assess how tumor clonality was changed by nivolumab, the variant clonality was assessed. In pretreatment samples, clonal and subclonal abundance was not associated with survival, while on-therapy clonal abundance was associated with worse outcomes. To determine how clonality shifts occur during nivolumab treatment in responders, distribution of CCF values was analyzed for pre- and on-treatment variants. This revealed that variants belonging to clones with a higher mean clonal fraction were more likely to undergo contraction in responders than in non-responders.
Clonal trajectory data showed selective clonal pruning during nivolumab treatment. Therefore, a neoantigen screen was performed focused on selecting the candidate neoantigens that were reduced on therapy. For 14 patients, blood was collected before and during treatment, and CD8+ T cells were screened using combinatorial tetramer screening. Out of 1,453 peptides that were selected for the screen, 502 could bind to patient-specific MHC class I alleles and produce tetramer (binders). From these, 196 formed tetramers that were recognized by CD8+ T cells (tetramer+/immunogenic). Of all predicted neoantigens, 5% were found to be immunogenic.
Analysis of the abundance of tetramer+ T cells over time showed that the tetramer+ peptides were more likely to be associated with increased T cells on-therapy, which was shown in all treatment groups, and was not significantly different between response groups. Neoantigens based on mutations that had a larger decrease in CCF during therapy were more likely to have an increase in related neoantigen-specific T cells before and during treatment than those with smaller changes. However, the presence of neoantigen-specific T cells did not guarantee selection against the neoantigen.
In patients with PR and SD, there was a higher frequency of binders and tetramer+ peptides than in those with PD. Clonal evolution was evaluated concurrently with T cell tetramer-positivity status, and this revealed that tetramer+ peptides were more likely to originate from a somatic variant that had a reduction in CCF on therapy. Tetramer+ T cells and on-treatment evolution mostly targeted clonal mutations, and the relationship between tetramer+ T cells and tumor evolution was only present in responders.
Since biophysical characteristics of MHC-presented peptides can increase the efficiency of T cell receptor (TCR) engagement, the researchers assessed what neoantigen properties make them more likely to bind MHC class I molecules and be tetramer+. Using machine learning, biophysical properties of immunogenic peptides per position were determined. NetMHCPan scores were found to be the most important feature, and amino acid index features relating to positions 2, 4, and 9 were highly enriched in immunogenic peptides. The lasso model was used to score peptides in the test data and validation sets of curated cancer neoantigens. Comparing these experimentally validated cancer neoantigens showed that features selected by the lasso model were enriched in immunogenic peptides.
After identifying features of immunogenicity, Alban and Riaz et al. determined whether these features could help predict immunotherapy response. Initially, no association between neoantigens and therapy response was observed. They selected the top one-third of scored peptides from the lasso model as potential immunogenic peptides. Variants in the same clone as predicted tetramer+ peptides were much more likely to be reduced on therapy.
The researchers then focused on HLA-A*02:01 for a subset analysis (n=4). To identify biophysical properties contributing to immunogenic peptides, peptide features unique to tetramer+ peptides of HLA-A*02:01 were extracted. This resulted in a ranked list of amino acid features at peptide positions that were enriched in tetramer+ peptides, and showed that features at position 4 were most enriched. Increased mutability, the evolutionary likelihood of an amino acid to accept a substitution in a source protein, at position 4 occurred in a region also known to allow for amino acid exposure to the TCR when bound to HLA. A multivariable linear regression model was developed in which the total number of tetramer+ T cells for a given binder was set as the response variable. This identified significantly different scores between tetramer- and tetramer+ peptides. The immunogenicity score was significantly different between non-binders and tetramer- peptides, suggesting this approach could enrich for immunogenic peptides.
The data in this study reveal more information on the neoantigen landscape and therapy-induced immunoediting of the tumor in response to nivolumab treatment. Further studies may help predict and further define what constitutes a successful antitumor T cell response to ICB treatment.
Write-up by Maartje Wouters, image by Lauren Hitchings
Meet the researcher
This week, co-first author Tyler Alban answered our questions.
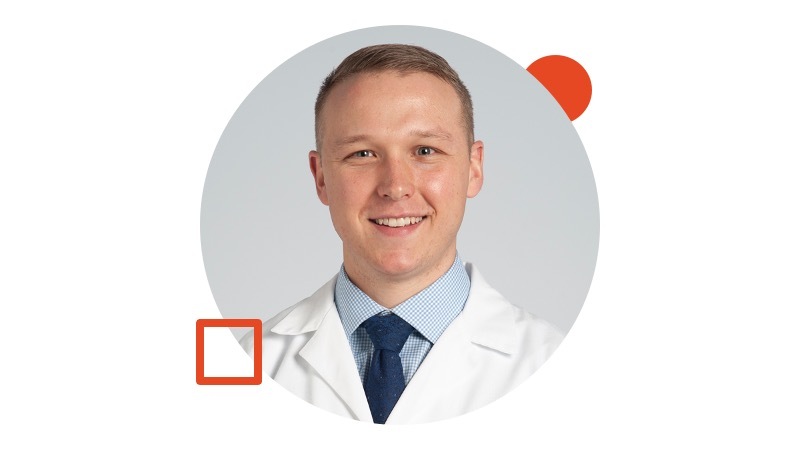
What was the most surprising finding of this study for you?
Here we focused on better understanding immunotherapy-induced neoantigen responses; however, neoantigens are usually patient-specific, T cell responses are patient-specific, and neoantigen prediction tools are unreliable for predicting immunogenicity. To overcome these limitations, we developed a large-scale neoantigen screen of 14 patients and >1,400 neoantigens. In addition, we tested both HLA binding and immunogenicity separately so that we could capture features that distinguished HLA binding from an immunogenic response. Together this allowed us to develop a new machine learning tool that added features from HLA binding and features from immunogenicity together to help us identify features driving immunogenicity. One of the most surprising findings was that patients who did not respond to immunotherapy still had neoantigen targeting T cells, but they appeared to target more subclonal populations.
What is the outlook?
This study provides a unique dataset for understanding the features that distinguish HLA binding from T cell receptor recognition of cancer neoantigens. We are pushing forward with this dataset, developing new tools for immunogenicity prediction in a collaboration with IBM.
What was the coolest thing you’ve learned (about) recently outside of work?
When I’m not programming I like to spend my time mountain biking and camping.